PHILADELPHIA (November 22, 2021)—Fox Chase Cancer Center has begun using a new artificial intelligence-powered system called AlphaFold, which allows researchers to develop accurate predictions of how proteins fold into their final structures, making it a tool that could enhance the development of new drugs.
Proteins are complex structures made up of amino acids. Their function ultimately depends on their final 3D shape, making knowledge of their 3D form paramount to a clearer understanding of how they work in the body.
How a protein’s amino acid sequence determines a protein’s shape has long been referred to by scientists as “the protein-folding problem,” given the vast number of ways a protein can fold before settling into its final shape.
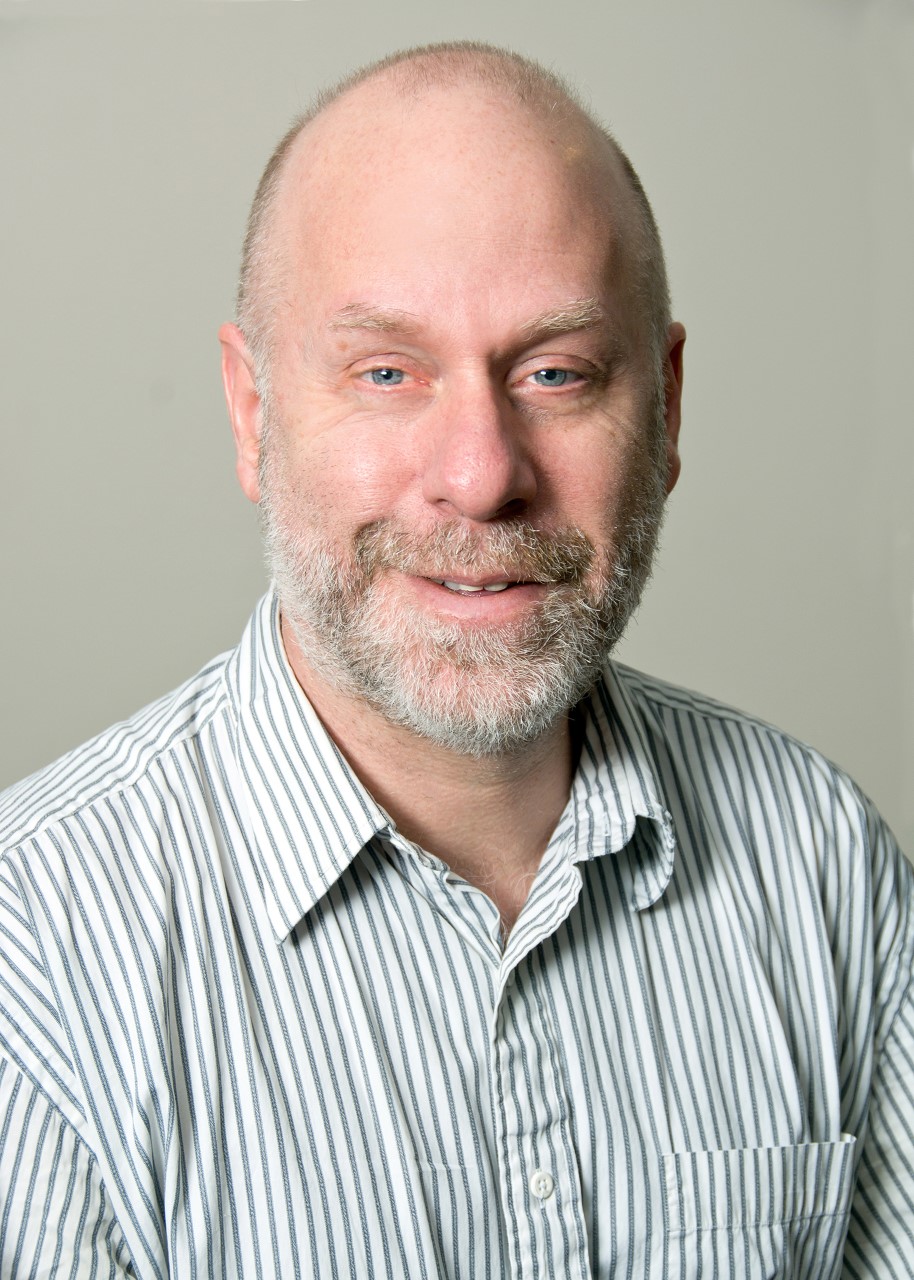
“What DeepMind did is develop a deep-learning algorithm. It’s a mathematical and computational algorithm to take huge amounts of data and use it to predict things. The most common use for this kind of method is image recognition,” said Roland L. Dunbrack Jr., PhD, professor and director of the Molecular Modeling Facility at Fox Chase.
“DeepMind has trained a neural network to take all of the 3D structures of proteins in the Protein Data Bank—about 180,000 structures and their sequences—and output a predicted structure of the protein in the form of three-dimensional coordinates,” Dunbrack said.
DeepMind is a scientific discovery company founded in 2010 and is part of Alphabet, the parent company of Google. According to the company, its mission is to “solve intelligence to advance science and benefit humanity.” Its accomplishments include AlphaFold, AlphaGo, more than 1,000 published research papers—including over a dozen in the journals Nature and Science—partnerships with scientific organizations, and hundreds of contributions to Google products.
In July, DeepMind partnered with the European Molecular Biology Laboratory, Europe’s flagship laboratory for the life sciences, to make the most complete and accurate database yet of the human proteome freely and openly available to the scientific community through the AlphaFold Protein Structure Database. The human proteome comprises the approximately 20,000 proteins expressed by the human genome. The company also published the system’s full methodology and open source code in Nature.
AlphaFold garnered attention last December at the 14th Critical Assessment of Protein Structure Prediction, an annual meeting and experiment held by the Protein Structure Prediction Center to identify progress being made in the field of protein structure prediction. During the meeting, AlphaFold vastly outperformed other methods of protein structure prediction in blind tests, Dunbrack said.
“What we can do with AlphaFold, specifically in cancer, is predict the structure of the protein in which a mutation occurs. We can see where that mutation is, see how the mutated protein interacts with other molecules, and ultimately get an idea about the mechanism, or why that mutation causes a problem for that cell. Once you have a mechanism for how a mutation causes a problem, you can begin to think about strategies for stopping that problem,” he said.
Dunbrack used it recently to predict how the breast cancer associated protein BRCA1 interacts with one of its partners during DNA repair. “Coincidentally, an experimental structure of the complex of these two proteins came out just after we performed the structure predictions. AlphaFold’s model was spot on,” he said.
Fox Chase had been using the structure predictions generated by AlphaFold, but at that time the available system did not have the ability to set all the operational parameters available with the new full system, which Dunbrack now has operating on computers at Fox Chase.
“It’s a very exciting time for us because we can do things that we just couldn’t do a couple of months ago. There are proteins people have approached us with in the past that they’re interested in, and we just can’t give them an answer because there are no experimental structures close enough in sequence to their protein of interest,” said Dunbrack.
“It was sometimes frustrating because they’re working on an important pathway to cancer treatment and they need to know more about what a certain component looks like structurally and how it works. We haven’t been able to give them an answer, but now we can,” he added.