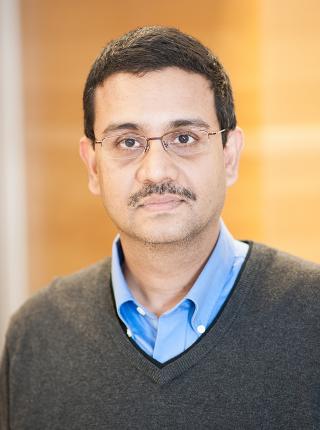
PHILADELPHIA (June 10, 2020)—In a recently published study, researchers at Fox Chase Cancer Center outlined new methods for handling data from large-scale studies that generate genomic profiles, with the goal of predicting survival rates and outcomes for patients being treated for cancer.
Genomic profiling is a process used to understand the way genes interact with each other and the environment of a specific individual. It can be used to develop new methods of monitoring, diagnosing and preventing diseases, including cancer, by identifying specific genomic features.
“Our approach provides an alternative, clinically useful explanation of risk in terms of odds of death, disease progression, or recurrence. It enables short-term and long-term risks to be combined into a single measure that quantifies the effect of a genomic feature on a patient’s survival outcome,” said Karthik Devarajan, PhD, an associate professor of population science in the Biostatistics and Bioinformatics Facility at Fox Chase.
These methods are critical in predicting outcomes, particularly because there are several challenges researchers face when using standard statistical methods in analyzing and interpreting the effect of genomic profiles on cancer outcomes.
“In some cases, when patients are monitored over time, a subject may leave the study prematurely or the event of interest may not occur during the study period, resulting in censored information. The number of such genomic markers of interest also far outnumbers the subjects that can be followed up clinically,” he said.
These factors make it difficult to apply standard statistical methods such as the Cox proportional hazards model, which is used to estimate the association between survival time and genomic features. The model assumes that each genomic feature of a tumor results in a constant risk of death, disease progression, or recurrence over the entire period of follow up in a study.
“Such an assumption is not only unverifiable but also unlikely to actually hold true for each feature. Moreover, there is empirical evidence indicating that the effect of some features increases or decreases the risk over time,” said Devarajan. “A review of the cancer literature revealed that only 5 percent of all studies actually checked this assumption and an even smaller fraction of clinical trials reported verifying it.”
These incorrect assumptions can lead to inaccurate conclusions that underestimate or overestimate the effect of a genomic feature. This could mean that a significant feature could be missed while a less significant one could be wrongly declared as being important in predicting survival. For this reason, it is important for statistical methods to be able to distinguish between features that suggest a constant risk of death or recurrence from those that have a time-varying effect.
“Our methods are designed to handle the large volume of genomic data, incorporate censored information, account for confounding clinical factors, and relax the fundamental assumption of constant impact of a genomic feature on a patient’s risk over time,” said Devarajan.
He added that by accurately identifying genomic markers associated with survival outcomes, their work could aid in the design of clinical studies that could ultimately lead to improved and personalized cancer treatments.
The study, “Unified Methods for Feature Selection in Large-Scale Genomic Studies With Censored Survival Outcomes,” was published in the journal Bioinformatics.